AI is one of today's most discussed technologies, set to transform industries and unlock opportunities. Businesses everywhere see its potential for boosting efficiency and growth and gaining a competitive advantage.
But making the most of AI isn’t straightforward and demands a strategic approach.
We often hear that organizations chase AI solutions for problems that don’t need such advanced methods. This leads to scattered, gimmicky projects that don't offer real value. For AI to be effective, it should align with a company's core goals and be driven by business insights, not just available data.
We also see companies jumping into AI initiatives without laying a strong foundation, which is crucial for success.
Consider a B2B company where the leadership aims to use AI to shake up its growth strategy. The management team imagines advanced models that adapt to market dynamics and drive more informed decision-making. But as they dive into it, they face two issues:
They don’t have enough data, in volume and granularity
It is a misconception to think we live in a big data world, oftentimes we just don’t. Within high-level segments over 10,000 records are needed to run decent AI and get meaningful insights.
Their data is fragmented across multiple systems
It's not uncommon for companies that have grown through acquisitions in the past to find themselves managing several systems across entities. The data scattered across platforms is leading to time-consuming, manual consolidations of the financial or customer that take days each month and thousands of hours annually.
Without first addressing its tech and analytics groundwork, the company’s ambitious AI plan fails. Focusing on essentials like data automation, dynamic reporting, and performance insights is crucial before jumping into AI-driven solutions, which aren’t always the immediate answer.
The importance of a solid foundation
AI models are only as good as the data fed into them. While AI has the potential to optimize processes in the future, it requires upfront investment in data and analytics infrastructure. To fully utilize AI, companies must first lay a robust groundwork in data management and analytics, covering key areas such as:
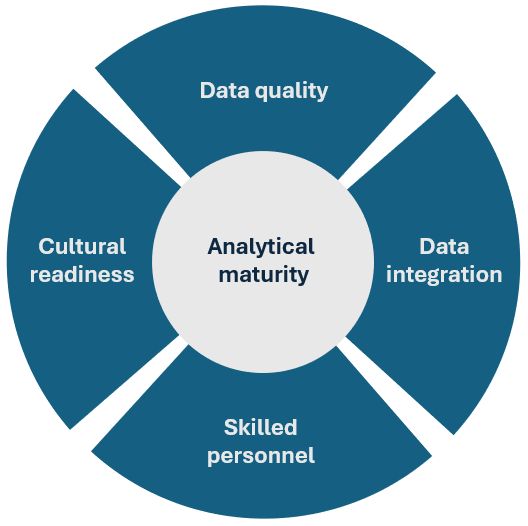
Data quality: Ensuring data accuracy, consistency, and integrity. Strong data governance policies help maintain high-quality data, which is crucial for reliable AI outcomes.
Data integration: Data silos across departments often limit insights. Integrating these data sources provides a holistic view, which is essential for accurate and meaningful analysis.
Analytical maturity: Organizations must evolve from descriptive analytics (understanding what happened) to diagnostic analytics (understanding why it happened), and eventually to predictive and prescriptive analytics (forecasting future trends and advising on actions).
Skilled personnel: A skilled team—including data scientists, data engineers, and domain experts—is essential for developing and implementing effective AI solutions.
Cultural readiness: The organizational culture should value data-driven decision-making, fostering an environment where insights from data are both trusted and acted upon.
Introducing our maturity model
Before jumping into AI initiatives, a company should first assess its current maturity level and define its target state. At Chronion, we use a structured maturity model that helps businesses progress through the critical steps of data management and analytics before delving into AI. This model provides a roadmap to assess existing capabilities, identify improvement areas, and guide companies toward their next level of maturity.
Our maturity model categorizes companies into one of four stages:
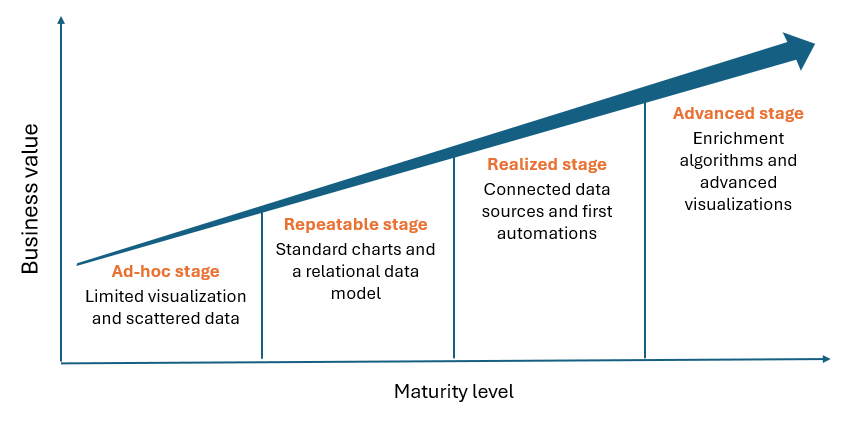
Ad-hoc stage: At this stage, companies lack structured analytics capabilities and primarily use basic tools like Excel to manage data.
Repeatable stage Here, companies begin to integrate data sources, utilizing standard reporting tools and self-service analytics to support more consistent analysis.
Realized stage: Companies in this stage detect and act on business opportunities through data insights, using descriptive and diagnostic analytics to inform decisions.
Advanced stage At this stage, companies leverage AI and machine learning, applying predictive and prescriptive analytics to inform forward-looking business strategies.
Each stage builds on the previous, rather than replacing it. Starting from the basics and gradually advancing ensures a solid foundation that supports the long-term adoption of AI.
The journey to effective AI implementation is a marathon, not a sprint. By focusing on building robust data and analytics capabilities, your organization will be well-prepared to unlock the full potential of AI and drive lasting business impact.
If you're interested in learning more or in developing a step-by-step strategy to advance your company’s analytical maturity, reach out to us. We're here to help guide you through a successful AI journey.
About the author
Pauline Stas is senior consultant at Chronion.
Dries Debbaut is partner at Chronion.
Do you want to know more about AI & digital transformation, contact us.
Comments